What if we could just ask AI to be less biased?
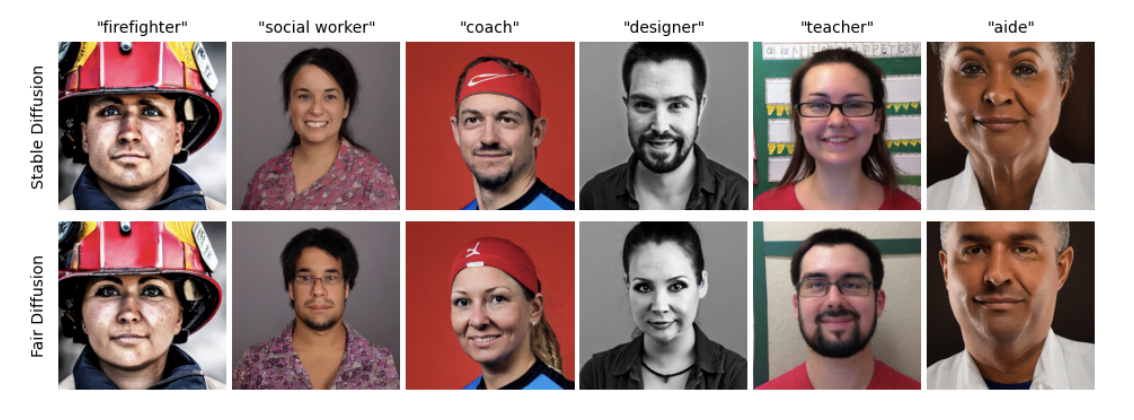
This method lets people create the images they want without having to undertake the cumbersome and time-consuming task of trying to improve the biased data set that was used to train the AI model, says Felix Friedrich, a PhD student at TU Darmstadt who worked on the tool.
However, the tool is not perfect. Changing the images for some occupations, such as “dishwasher,” didn’t work as well because the word means both a machine and a job. The tool also only works with two genders. And ultimately, the diversity of the people the model can generate is still limited by the images in the AI system’s training set. Still, while more research is needed, this tool could be an important step in mitigating biases.
A similar technique also seems to work for language models. Research from the AI lab Anthropic shows how simple instructions can steer large language models to produce less toxic content, as my colleague Niall Firth reported recently. The Anthropic team tested different language models of varying sizes and found that if the models are large enough, they self-correct for some biases after simply being asked to.
Researchers don’t know why text- and image-generating AI models do this. The Anthropic team thinks it might be because larger models have larger training data sets, which include lots of examples of biased or stereotypical behavior—but also examples of people pushing back against this biased behavior.
AI tools are becoming increasingly popular for generating stock images. Tools like Fair Diffusion could be useful for companies that want their promotional pictures to reflect society’s diversity, says Kersting.
These methods of combating AI bias are welcome—and raise the obvious question of whether they should be baked into the models from the start. At the moment, the best generative AI tools we have amplify harmful stereotypes on a large scale.
It’s worth remembering that bias isn’t something that can be fixed with clever engineering. As researchers at the US National Institute of Standards and Technology (NIST) pointed out in a report last year, there’s more to bias than data and algorithms. We need to investigate the way humans use AI tools and the broader societal context in which they are used, all of which can contribute to the problem of bias.
Effective bias mitigation will require a lot more auditing, evaluation, and transparency about how AI models are built and what data has gone into them, according to NIST. But in this frothy generative AI gold rush we’re in, I fear that might take a back seat to making money.