Effective infrastructure enables universal data intelligence
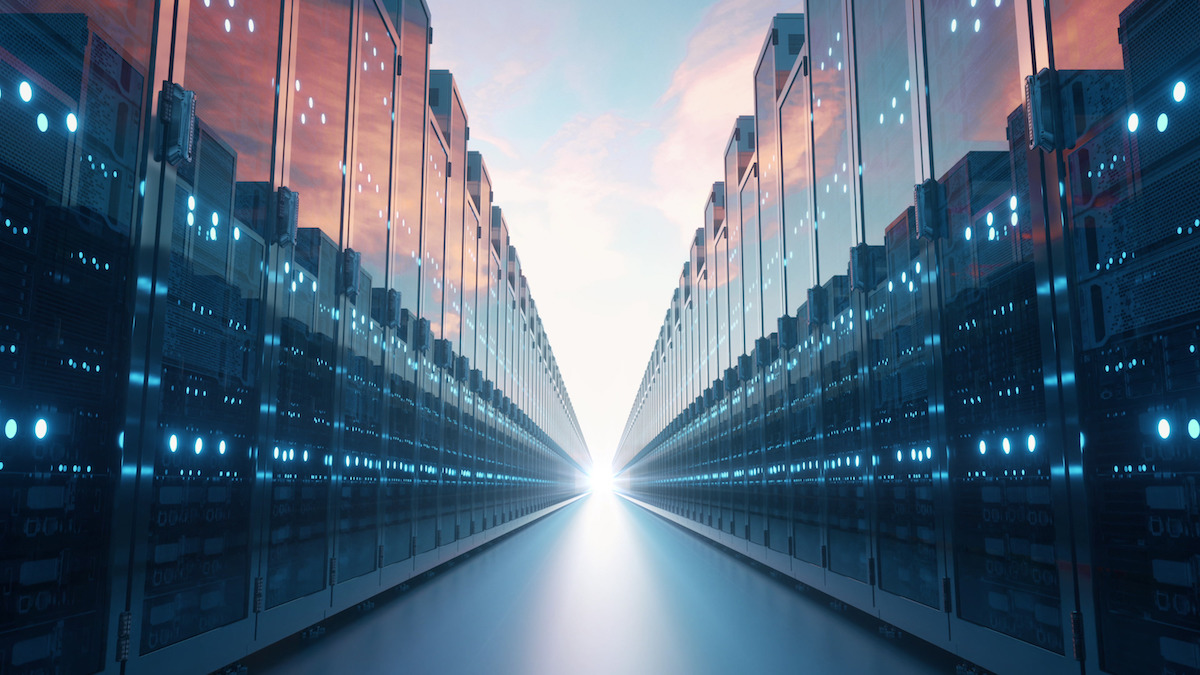
As organizations transition to data-driven business models, they become keepers of immense and ever-growing amounts of data, which they must store, protect, and analyze. For many this is a sizable challenge: 80% of respondents to a survey by 451 Research said they work with more than 100 data sources. Their existing systems also struggle to keep pace: 30% of respondents said it takes their organization more than a week to go from data to insights.
Consequently, most organizations face a long list of data-related challenges. They must get their arms around a massive volume of data, ranging from historical to real-time. They need to determine what type of infrastructure modernization is required to process all that data, and then how to integrate that storage infrastructure with data services, workloads, and applications. And the questions continue: How do we apply automation, AI, and machine learning to data sets? How should we think about the cloud? And how do we take advantage of as-a-service models to deliver data-driven value to the business?
And above all, today’s economic conditions call for doing more with less. How can IT teams meet the business need for advanced data analytics when their budget growth is not keeping pace?
First steps toward data intelligence
Bharti Patel, senior vice president of product engineering at Hitachi Vantara, says businesses should aspire to offer seamless access to data and insights, what she calls “universal data intelligence.” That journey starts with getting a handle on the basics of data discovery and data classification and creating policies for how the organization handles different types of data.
“Thoughtfulness at the beginning of the journey is very important,” says Patel. To derive insights with business value, organizations first must get a handle on the data they have and are collecting. “People really don’t fully understand what value lies in their data; they don’t know where it resides or how to make the best use of it,” she says. “Your strategy about what data goes where—what goes to tape, what stays on-prem, what goes to cloud—is very important.”
To create that strategy, organizations need to define the purpose of their data: Is this data we’re storing simply for compliance purposes, with a low probability that we will ever need to retrieve it? Is this confidential data that must be stored on-premises, with multiple backups? Or is this data that needs to be accessed frequently and should be stored on high-performance NVMe (non-volatile memory express) systems?
Organizations must also prioritize data analytics initiatives to find the right balance between quick-hit projects that deliver limited benefit against more ambitious endeavors that could take longer but deliver more value in the long run.
Patel says it’s vitally important that organizations establish clear lines of communication between business and IT leaders. Everyone should be on the same page when it comes to identifying the most pressing business needs, agreeing on a priority list, and making sure that deliverables from the data analytics teams are presented in a way the business can put to use.